In this article, we briefly introduce the reader to the KAPSARC Toolkit for Behavioral Analysis (KTAB). KTAB is a toolkit for building models of collective decision-making processes (CDMP), and simulating them. KTAB is being developed by the King Abdullah Petroleum Studies and Research Center to meet the need for widely available, state-of-the-art, supported, transparent, and open-source software that facilitates the modeling and analysis of CDMPs. We summarize insights from two case studies modeled with KTAB, in which important policy questions in the Arab Gulf region were modeled using two different forms of CDMP models. We document the two forms of quantitative CDMP models used, both based on the informal exertion of influence. This is an important set of models, because actual votes cast in many situations are ultimately the result of prior informal negotiation. The Spatial Model of Politics is a powerful tool for the political analyst modeling policy questions which can be represented as a multidimensional continuous spectrum. However, not all policy questions can be represented in this manner. The Probabilistic Condorcet Election is a second, and completely general, form of CDMP model that imposes almost no structure on the problem analyzed. © 2017 Association for Computing Machinery.
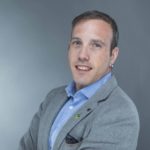